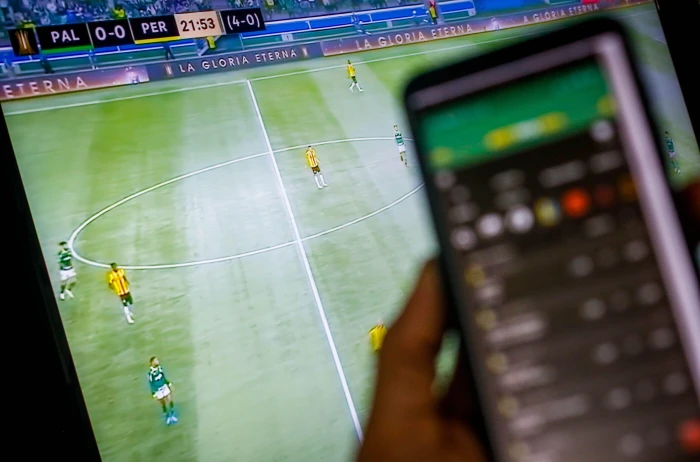
Mathematical models have transformed what was once considered gambling into a sophisticated investment strategy in the evolving landscape of sports betting. Modern bettors are increasingly adopting advanced mathematical approaches to gain an edge in a market where bookmakers have traditionally dominated for decades.
The Evolution of Sports Betting Mathematics
Sports betting has historically relied on odds set by bookmakers, with simple probability concepts guiding most wagers. However, the introduction of computational power, big data analytics, and statistical modeling has revolutionised the industry. Today's successful sports bettors employ complex mathematical frameworks that extend beyond basic probability.
The mathematical evolution began with John L. Kelly Jr.'s 1950s development of the Kelly Criterion. This formula determines the optimal size of sequential bets to maximise long-term growth while minimising the risk of ruin. While this remains foundational, contemporary approaches have expanded considerably upon these early concepts.
Expected Value: The Cornerstone of Profitable Betting
At the heart of modern sports betting mathematics lies the concept of expected value (EV). This fundamental calculation determines whether a bet offers positive long-term value:
EV = (Probability of Win × Amount Won per Bet) - (Probability of Loss × Amount Lost per Bet)
When the EV is positive, a bettor has identified an opportunity with a mathematical advantage. However, the challenge lies in accurately calculating the true probability of an outcome, which often differs from the implied probability represented in bookmakers' odds.
Predictive Modeling and Machine Learning Applications
Advanced bettors now develop sophisticated predictive models incorporating machine learning algorithms that process vast data. These models consider variables including:
- Historical performance data
- Team and player statistics
- Venue information and home-field advantage
- Weather conditions
- Injury reports
- Coaching tendencies
- Public betting patterns
The AI-powered machine learning applications in sports betting utilise neural networks, random forests, gradient boosting, and other algorithms to identify patterns that human analysts might miss. These systems continuously improve their predictions through iterative learning processes, adapting to new information and refining accuracy.
Bayesian Statistics in Sports Betting
Bayesian statistical methods have gained prominence in sports betting mathematics. Unlike traditional statistical approaches, Bayesian analysis incorporates and updates prior beliefs based on new evidence. This approach is particularly valuable in sports betting, where circumstances constantly change.
The Bayesian framework allows bettors to:
- Formulate initial probabilities based on prior knowledge
- Update these probabilities as new information emerges
- Calculate posterior probabilities that reflect the most current understanding
- Make decisions based on these updated probabilities
This methodology excels in adapting to dynamic situations, such as in-play betting, where odds shift rapidly as games progress.
Arbitrage and Line Shopping Mathematics
Mathematical arbitrage represents a risk-free profit opportunity. It involves placing bets on all possible outcomes of an event at odds that guarantee a profit regardless of the result. While pure arbitrage opportunities have become increasingly rare due to bookmaker vigilance, the underlying mathematics still applies to "value arbitrage," which involves finding significantly mispriced odds across different bookmakers.
Line shopping, the practice of comparing odds across multiple bookmakers, has been quantified mathematically. The expected value gain from consistently finding superior odds can be substantial over time:
Closing Line Value (CLV) = (Odds Obtained / Closing Odds) - 1
Positive CLV indicates that a bettor secured better odds than the final market consensus, which predicts long-term profitability.
Regression Analysis and Sports Modeling
Regression analysis helps quantify relationships between variables that affect sporting outcomes. Multiple regression models can identify which factors most significantly influence results. For example, in basketball betting, regression analysis might reveal the statistical weight of three-point shooting percentage versus rebounding in determining game outcomes.
These models generate point spreads or total scores that can be compared against bookmaker lines to identify value opportunities. A potential betting opportunity exists when a model's projection differs significantly from the market line.
Ensemble Methods and Consensus Models
The team at PlayMichigan told us that “Successful sports bettors increasingly employ ensemble methods that combine multiple models rather than relying on a single mathematical approach.” This methodology, borrowed from data science, reduces variance and improves prediction accuracy by leveraging the strengths of different modeling techniques.
A typical ensemble might include:
- Statistical regression models
- Machine learning algorithms
- Power rating systems
- Public consensus data
- Expert opinion weightings
By synthesising these diverse approaches, bettors develop more robust predictions that are less susceptible to the weaknesses inherent in any single model.
Risk Management Mathematics
Sophisticated sports bettors implement portfolio theory concepts borrowed from financial markets to optimize their betting strategy. This mathematical framework addresses:
- Bet sizing based on edge magnitude
- Bankroll allocation across multiple wagers
- Correlation between bets
- Variance reduction techniques
- Maximum drawdown tolerance
The Kelly Criterion, mentioned earlier, often serves as a starting point. Still, modern approaches typically employ fractional Kelly (betting a percentage of the Kelly-recommended amount) to reduce variance while maintaining positive expectations.
Market Efficiency and Closing Line Theory
The mathematics of market efficiency plays a crucial role in sports betting strategy. According to closing line theory, the final odds before an event starts represent the most accurate assessment of true probabilities as they incorporate all available information and betting activity.
Bettors who consistently secure odds better than closing lines demonstrate an ability to identify value before the market consensus. The mathematical analysis of this "closing line value" is a key performance indicator for long-term profitability.
Poisson Distribution in Low-Scoring Sports
The Poisson distribution provides a mathematical framework for modeling expected goals and game outcomes for sports with relatively low scores, such as soccer and hockey. This probability distribution expresses the likelihood of events occurring within a fixed interval when they happen independently at a constant average rate.
The formula allows bettors to calculate the probability of specific scorelines and total goals, offering insights into the value of various betting markets beyond simple match results.
The Mathematics of Market Inefficiencies
Unlike financial markets, sports betting markets contain persistent inefficiencies that can be exploited mathematically. These include:
- Recreational bettor biases toward favourites and overs
- Overreaction to recent performance
- Improper weighting of key statistics
- Regional betting preferences
- Limited market liquidity in secondary markets
Quantitative analysis can identify and exploit these inefficiencies through systematic rather than intuition-based betting.
Significance Testing in Betting Systems
Determining whether a betting system genuinely works requires rigourous statistical testing. P-values, confidence intervals, and sample size calculations help distinguish between genuine edges and results attributable to random variance.
The mathematics of significance testing prevents bettors from being misled by small sample sizes or selective endpoints, issues that plague many commercial betting systems lacking mathematical validity.
The Future of Betting Mathematics
The integration of sports betting mathematics continues to evolve with advancements in computing power, data availability, and algorithmic sophistication. Emerging areas include:
- Real-time player tracking data analysis
- Computer vision applications
- Natural language processing of news and social media
- Quantum computing applications for complex modeling
- Blockchain-based prediction markets
The gambling experts at PlayMichigan estimated that these developments promise to further refine the mathematical foundations of profitable sports betting strategies
The Mathematical Edge
The mathematics behind profitable sports betting has transformed from simple probability calculations to a sophisticated discipline incorporating elements of statistics, computer science, economics, and game theory.
As sports betting markets expand globally, the mathematical sophistication required to maintain an edge will only increase. The most successful bettors will be those who continuously refine their mathematical models, adapt to changing market conditions, and maintain disciplined implementation of their quantitatively derived betting data.